1) FAIRVASC has released a new data quality analysis lately. Can you comment on it?
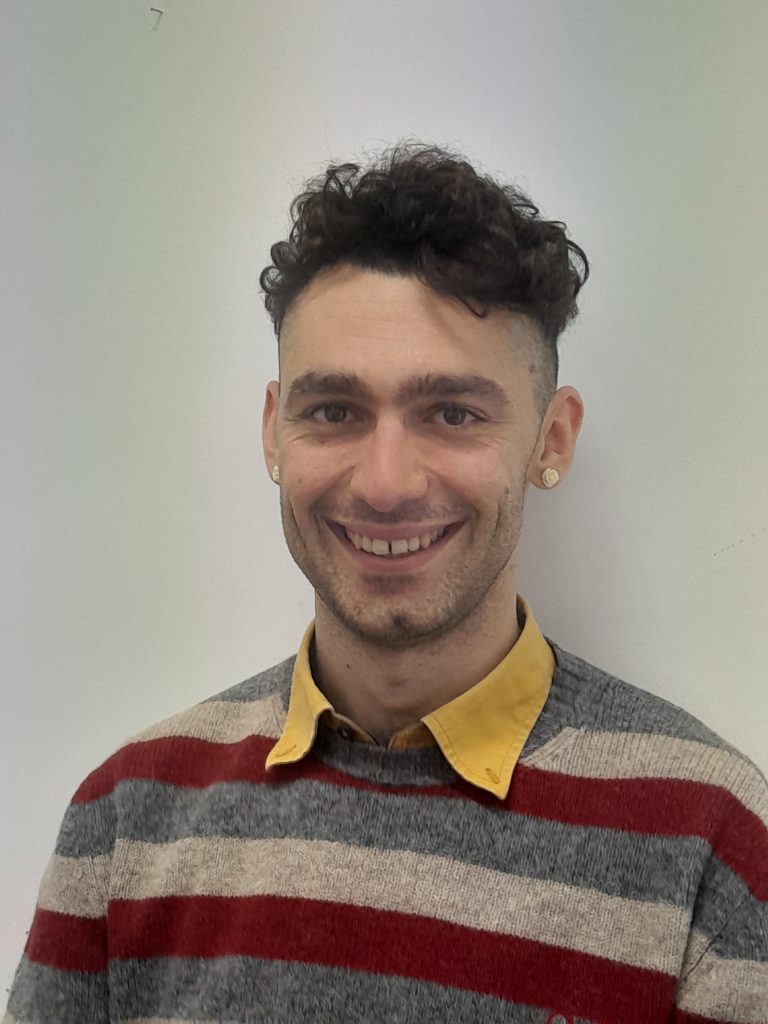
Major differences with respect to the previous run are: 1. the addition of two new variables (namely CRP at diagnosis and induction treatment) amongst the ‘DQ core variables’, and 2. the stratification of the DQ analysis based on the diagnosis. Moreover, we decided to collect the results for each variable as absolute numbers rather than percentages. This way, it will be easy to calculate percentages at a later stage based on the sub-cohort we will want to focus on (e.g. if we want to get the data quality statistics for the GPA+MPA population, then it will suffice to go and look at the absolute numbers for the GPA and MPA patients and do the calculations). We measured scores close to 100% across all registries and for all variables with regard to the uniqueness of the data and to the consistency of the data (including also logic tests and plausibility). Completeness of the data is quite good as well, while the correctness is still to be evaluated in this present second run of the DQ analysis.
2) Could you point out a few most important pieces of advice you would give to improve data quality in registries.
The DQ analysis itself is a tool that allows registries to spot errors and to consider a re-entry of inconsistent data (assuming that the data source – usually the clinical record of the patient – can be retrieved). The DQ improvement can be prospective as well: the usefulness of the DQ analysis lies in the opportunity to identify the weak points of the registry data and to investigate them to understand their root source. For example, after detecting an elevated missingness in one of your variables, you might search and find out that it’s due to the way the personnel in charge enter that kind of data. And this could inspire the registry team to organise an event dedicated to awareness creation, intended to the personnel in charge of entering the data in the clinical record. This could be a strategy to prospectively improve the data that will be included in the registry.
3) What are the next steps in data quality analysis that FAIRVASC is going to take?
This is still to be discussed with the team, but there are some options. One is to repeat the DQ analysis, but this time choosing the ‘DQ core variables’ such that they match the variables that are actually used in the queries posed with the interface. Another possibility is to increase the cross-variable checks: we already included some logic tests in our DQ analysis that compare the values of two variables (for example “is date of death later than date of birth?”) rather than just evaluating the presence/absence of the value or its consistency/plausibility. We could add some further tests like these as an additional layer of DQ assessment.